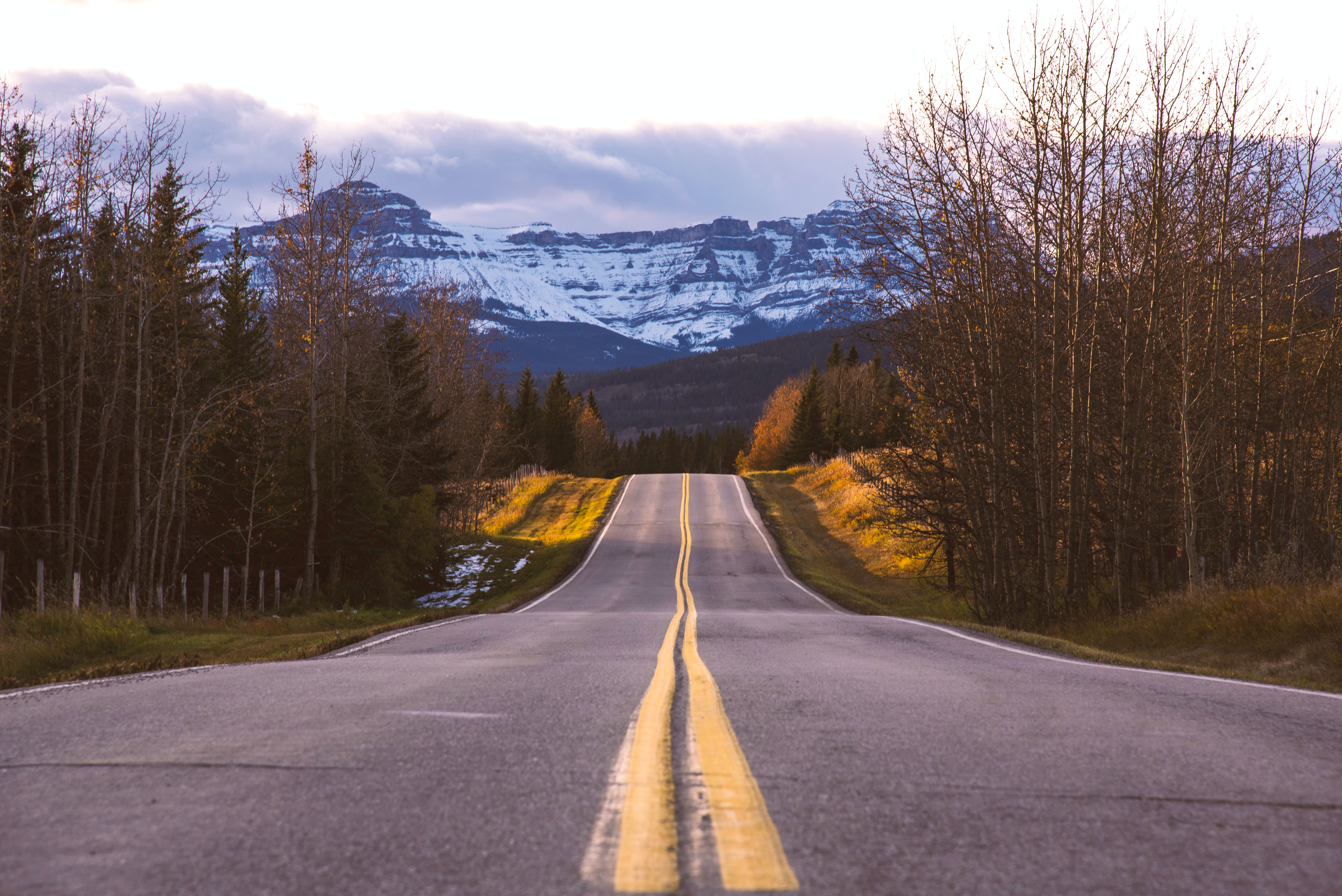
You’ve got a business goal in mind that you think AI might be able to help with. Or you could have ideas about potential new products, services, revenues, or customers if you could only solve the problem using AI. It might even be critical enough that your whole corporate future might rely on achieving. Or on the other end of the spectrum, it might be just an idea that you want to create a small pilot project to see where it might go.
You’re at A, and you want to get to B. “How do I get from A to B using AI?” is one of the most common questions I get asked by those who are looking to implement or adopt AI for the first time.
There is no silver bullet, but there is a yellow brick road. For Dorothy in the unknown and frightening land of OZ, as she tries to get to the Wizard to find a way home, the yellow brick road was her guide. In today’s techno world, the equivalent would be to “Follow the Data”. The wisdom is as simple – and as complicated – as that.
The need for data is at the roots of all the AI architectures and algorithms. AI researchers are always looking for data. They are data-hungry – the more, the better. The outcomes of what the networks learn are, in nearly all cases, totally data dependent – on both quantity and quality.
The primary reason why AI networks need more data is that they tend to be very large in the number of weights and can therefore overfit their learnings to the training data. As a result, they don’t generalise enough to be applied to similar but not the exact same data. On the other hand, if you make the network too small, it likely won’t learn the essential characteristics to make good decisions.
There is an old adage that “if you can’t measure it, you can’t control it.” That is all about data and really does have similarities to AI challenges. Having that in the back of your mind, whether from a business or engineering perspective, will provide helpful guidance to AI applications – and take some of the mystery out of it all.
Start with answering the questions like: What data do I already have? What data do I think I need? Where can I get data, inside or outside the company, to contribute to a significant dataset? What is the uniqueness of my data that no one else has?
Look to the entire company for ideas about data, not just the familiar places of engineering, IT, and product development. Your teams in marketing, finance, administration, and customer relations – and even the customers themselves – are excellent sources of ideas about data. You’d be amazed at what the company might have collected along the way.
One of the most important reasons to involve all your corporate teams is that they are the heart of your company. By having them involved right from the beginning in a new AI adventure, you’ll get their buy-in right from the start. It’s really easy as data is something they understand already and makes AI less frightening.
You might discover that you actually have terabytes of data without thinking too much. But is it what you really need? Is it good, clean data? How do you manage, sort, and organise the heaps of data you’ve got? And, if you have privacy issues, such as handling customer data, how do you protect it? That is where you need Data Scientist specialists. It has been said that three-quarters of an AI project is preparing the data even before the AI scientists get their hands dirty.
While on the topic of sayings, there is one from the early 1970s when computing was young: “Garbage In, Garbage Out!” Nothing could be truer than for AI. If the data you feed into the architectures is crap, don’t expect gold to come out.
Equally important is that there is the AI issue of “bias”. AI algorithms only learn from what they have been given. If your data has a bias in gender, race, culture, income, health, geography (…the list goes on), then your system will learn those bad traits too. Bias, ethics, and fairness are some of the most important AI research topics that are being explored today.
As a final note on the data journey, lots will be learned along the way. This will include how the heart of the company will engage and contribute; how well the corporate culture will learn and change to the new frightening world of AI with courage; and how all the company’s minds, not just those of your data and AI teams, will think about unique opportunities. Sure, there will be failures and twists along the way, but following the data “yellow brick road” is an excellent way to lead you to the “Emerald City” of AI success for the future.